A Federated Learning Approach for Type-2 Diabetes Detection Using a Naive Bayes Classifier
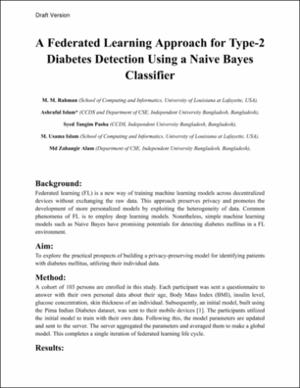
View/ Open
Date
2023-10Author
Rahman, M. M.
Islam, Ashraful
Pasha, Syed Tangim
Islam, M. Usama
Alam, Md Zahangir
Metadata
Show full item recordAbstract
Federated learning (FL) is a new way of training machine learning models across decentralized devices without exchanging the raw data. This approach preserves privacy and promotes the development of more personalized models by exploiting the heterogeneity of data. Common phenomena of FL is to employ deep learning models. Nonetheless, simple machine learning models such as Naive Bayes have promising potentials for detecting diabetes mellitus in a FL environment. To explore the practical prospects of building a privacy-preserving model for identifying patients with diabetes mellitus, utilizing their individual data. A cohort of 103 persons are enrolled in this study. Each participant was sent a questionnaire to answer with their own personal data about their age, Body Mass Index (BMI), insulin level, glucose concentration, skin thickness of an individual. Subsequently, an initial model, built using the Pima Indian Diabetes dataset, was sent to their mobile devices [1]. The participants utilized the initial model to train with their own data. Following this, the model parameters are updated and sent to the server. The server aggregated the parameters and averaged them to make a global model. This completes a single iteration of federated learning life cycle. Participants are diversed in gender: male (60.2%) and female (39.8%); in age groups: 20-35 (14.6%), 36-50 (46.6%), 51-65 (38.8%). The work shows an accuracy of 89.32% and a precision of 88.89% for those having diabetes while 90.32% precision in detecting patients not having a diabetes mellitus. The number of communication rounds was 50 where in each round at least two participants participants in building federated model updates. Since one of the key reasons for using FL is to improve data privacy, quantifying the level of privacy is critical. An network intruder could decoded the model updates by examining the changes in the global model over time. However, a membership inference attack (MIA) is measured in various differential privacy (DP) budgets. DP aims to prevent this kind of inference by adding noise to the data (model updates). For instance, if the model update would normally be a weight change of +0.5, a noise from a Laplacian distribution with mean 0 is added. Hence, the resulting noisy update might then be +0.52. A naive bayes based federated learning system is built to detect diabetes mellitus (Type-2) preserving the privacy of user data at the first place.
Collections
- 2023 [67]