Automatic Detection of Natural Disaster Effect on Paddy Field from Satellite Images using Deep Learning Techniques
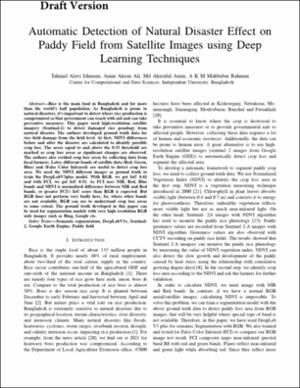
View/ Open
Date
2023-03Author
Alavi Ishmam, Tahmid
Ali, Amin Ahsan
Amin, Md Ahsraful
Rahman, A K M Mahbubur
Metadata
Show full item recordAbstract
Rice is the main food in Bangladesh and for more than the world’s half population. As Bangladesh is prone to natural disasters, it’s important to detect where rice production is compromised so that government can reach with aid and can take preventive measures. This paper used high-resolution satellite imagery (Sentinal-2) to detect damaged rice penology from natural disaster. The authors developed ground truth data for rice field damage from the field level. At first, NDVI differences before and after the disaster are calculated to identify possible crop loss. The areas equal to and above the 0.33 threshold are marked as crop loss areas as significant changes are observed. The authors also verified crop loss areas by collecting data from local farmers. Later, different bands of satellite data (Red, Green, Blue) and (False Color Infrared) are useful to detect crop loss area. We used the NDVI different images as ground truth to train the DeepLabV3plus model. With RGB, we got IoU 0.41 and with FCI, we got IoU 0.51. As FCI uses NIR, Red, Blue bands and NDVI is normalized difference between NIR and Red bands, so greater FCI’s IoU score than RGB is expected. But RGB does not perform very badly here. So, where other bands are not available, RGB can use to understand crop loss areas to some extent. The ground truth developed in this paper can be used for segmentation models with very high resolution RGB only images such as Bing, Google etc.
Collections
- 2023 [67]